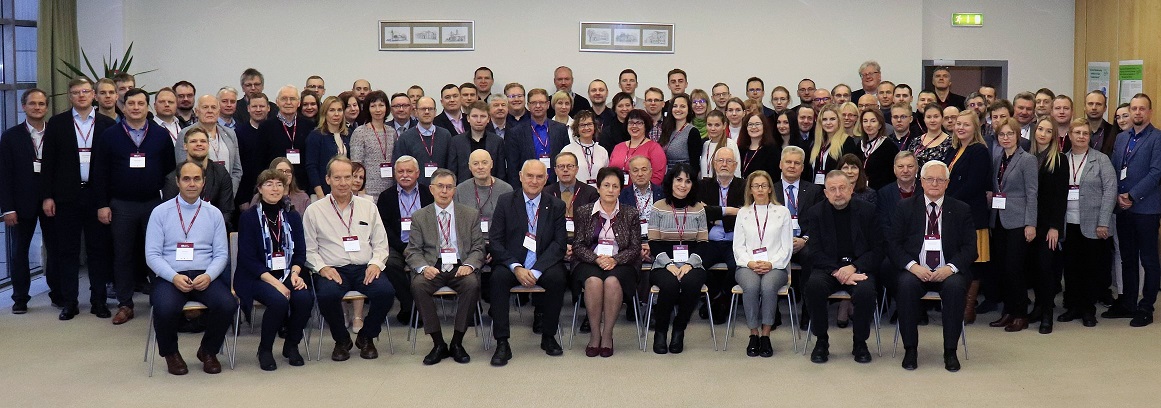
DAMSS 2019: Plenary Speakers
![]() |
Prof. Dr. habil. Juris Borzovs
Juris Borzovs is a founder and the first Dean of Faculty of Computing at the University of Latvia, currently – Vice-Dean. He is a Co-Editor-in-Chief of the Baltic Journal of Modern Computing. His research interests include software engineering methods and standards, software quality management, Latvian terminology of information and communications technology, information technology education and training. Juris Borzovs is a Corresponding Member of the Latvian Academy of Sciences, he has been awarded the Order of the Three Stars (Commander). |
Talk title: Do We Really Know How to Measure Software Quality?
Abstract: ISO/IEC 2502n series of standards that are devoted to system/software quality measurement currently consists of the following International Standards:
- ISO/IEC 25020 — Measurement reference model and guide: provides a reference model and guide for measuring the quality characteristics defined in ISO/IEC 2501n quality model division.
- ISO/IEC 25021 — Quality measure elements: provides a format for specifying quality measure elements and some examples of quality measure elements (QMEs) that can be used to construct software quality measures.
- ISO/IEC 25022 — Measurement of quality in use: provides measures including associated measurement functions for the quality characteristics in the quality in use model.
- ISO/IEC 25023 — Measurement of system and software product quality: provides measures including associated measurement functions for the quality characteristics in the product quality model.
Quality measure elements (e.g., number of faults) provide the base for measures of product and quality in use (e.g., functional correctness). The presentation examines practical completeness of the ISO/IEC 2502n series software product part.
![]() |
Prof. Dr. James M. Calvin
James Calvin is a professor in the department of computer science at the New Jersey Institute of Technology in Newark, New Jersey, USA. His work focuses on global optimization, in particular on average-case analysis of optimization algorithms. His interests include applications to computational problems such as image processing and clustering. He has also worked on stochastic simulation. He received a PhD degree in operations research from Stanford University. |
Talk title: Approximating the Minimum of a Smooth Gaussian Process
Abstract: Many of the difficult questions concerning global optimization are interesting and difficult even in the one-dimensional case, where they are more easily described. The purpose of this talk is to explore some of the natural questions that arise in the study of the average-case complexity of global optimization of smooth Gaussian processes. The reason for studying the average-case complexity of optimization is that if one optimizes over a convex and symmetric function class, nonadaptive algorithms are essentially as powerful as adaptive methods. For example, if the goal is to approximate the minimum of a function defined on the unit interval that is only known to be twice continuously differentiable, then evaluating the function at equi-spaced points is near optimal in terms of the worst-case error. In practice, adaptive methods are favoured, and while they can not be justified by their worst-case performance, adaptive algorithms have been shown to be much more efficient than nonadaptive methods on the average for some probability models. In this talk, we will consider the following type of question: Given an error tolerance ϵ > 0, on average how many evaluations of the function are required to obtain an expected error of at most ϵ? We will examine how the answer depends on the information available to the algorithm as well as the probability model.
![]() |
Prof. Andrzej Czyzewski
Prof. Andrzej Czyzewski, Ph. D., D. Sc., Eng. is a full professor at the Faculty of Electronics, Telecommunication and Informatics of Gdansk University of Technology. He is an author or a co-author of more than 600 scientific papers in international journals and conference proceedings. He has supervised more than 30 R&D projects funded by the Polish Government and participated in 7 European projects. He is also an author of 15 Polish and 7 international patents. He has extensive experience in soft computing algorithms and their applications in sound and image processing. He is a recipient of many prestigious awards, including a two-time First Prize of the Prime Minister of Poland for research achievements (in 2000 and in 2015). Andrzej Czyzewski chairs the Multimedia Systems Department in Gdansk University of Technology. |
Talk title: New Applications of Sound and Vision Engineering to Information and Communication Technology
![]() |
Prof. Emeritus Helen Karatza
Helen Karatza is a Professor Emeritus in the Department of Informatics at the Aristotle University of Thessaloniki, Greece, where she teaches courses in the postgraduate and undergraduate level, and supervises doctoral and postdoctoral research. Dr. Karatza's research interests include Computer Systems Modeling and Simulation, Performance Evaluation, Grid, Cloud and Fog Computing, Energy Efficiency in Large Scale Distributed Systems, Resource Allocation and Scheduling and Real-time Distributed Systems. Dr. Karatza has authored or co-authored over 220 technical papers and book chapters including five papers that earned best paper awards at international conferences. She is senior member of IEEE, ACM and SCS, and she served as an elected member of the Board of Directors at Large of the Society for Modeling and Simulation International. She served as Chair and Keynote Speaker in International Conferences. Dr. Karatza is the Editor-in-Chief of the Elsevier Journal “Simulation Modeling Practice and Theory” and Senior Associate Editor of the “Journal of Systems and Software” of Elsevier. She was Editor-in-Chief of “Simulation Transactions of The Society for Modeling and Simulation International” and Associate Editor of “ACM Transactions on Modeling and Computer Simulation”. She served as Guest Editor of Special Issues in International Journals. More info about her activities/publications can be found in http://agent.csd.auth.gr/~karatza/> |
Talk title: Scheduling Complex Applications in Cloud Systems – Challenges and Research Directions
![]() |
Assoc. Prof. Tatjana Loncar-Turukalo
Tatjana Loncar-Turukalo is an associate professor at Department of Power, Electronics and Telecommunication at the Chair of Telecommunications and Signal Processing, Faculty of Technical Sciences, University of Novi Sad, Serbia. She accomplished her PhD studies at the same institution. She teaches several subjects on signal processing and machine learning at Electrical Engineering and Biomedical Engineering. Her research interests are focused on biomedical signal processing and knowledge discovery from health-related data, the analysis and fusion of heterogeneous patient data for predictive modelling and identification of hidden dependencies. She aims at bridging the scales and merging information from cellular to phenotype level as enabling methodology for systems medicine and connected health. The recent application examples are gene-expression analysis, human microbiome, respiratory sound analysis, fMRI, and polysomnography data (arousal analysis). Tatjana has co-authored three book chapters and more than 90 publication in peer-reviewed journals and international conferences. She took part in many European and national research projects. She is an active member of two research centres at Faculty of Technical Sciences: Centre of Excellence CEVAS (The Centre for Vibro-Acoustic Systems and Signal Processing) and iCONIC (Centre for intelligent COmmunications, Networking and Information processing), and holds an IEEE, Engineering in Medicine and Biology, and Women in Engineering membership. |
Talk title: Data-driven Approaches for Predictive and Preventive Medicine
Abstract: As the worldwide population grows and the access to health care is increasingly being demanded, the need for a paradigm-shift towards pervasive, predictive and personalized health care decisions becomes evident. Connected health, a technology-enabled model of health management, relies on sensing, communications, and analytics, leveraging that technology to deliver more efficient and cost-effective health care models. The acquisition of health-related data from the patient in a pervasive and seamless manner, will allow for the use of powerful analytical tools not only in predictive purposes, but as well research wise to help understand the origins and silent progression of disease. There are still numerous obstacles to this aim mainly related to slow translation of discoveries in life sciences into more effective therapies, the lack of evidence in literature on advantages of connected health solutions, and only scattered efforts in interdisciplinary education in healthcare technologies. In this talk we would address some examples of data-driven approaches supporting medical decision making, from clinical level examples to the research on human microbiome and its association to disease. With an emphasis on challenges associated with different data types, the talk will offer some methodological approaches to tackle these problems, pointing out some important issues from a signal processing and machine learning perspective.
![]() |
Prof. Dr. Pilar Martínez Ortigosa
Pilar Martinez Ortigosa is a Full Professor of Architecture and Computer Technology at the University of Almeria, Spain. Her teaching activity is related to Computer Architecture and Technology, High Performance Computing, Computer Networks and Global Optimization. Her research has been focused on High Performance Computing (HPC), Metaheuristic Global Optimization, Multiobjective optimization, Evolutionary algorithms and the application to several real problems such as the alignment of images, reconstruction of images and competitive localization by participating in both the design of mathematical models that simulate real problems and metaheuristic optimization. Other relevant application line is devoted to the optimal design and working of Thermosolar Plants. Recently she is working in drug discovery problems, such as virtual screening procedures where the similarities of candidate new drugs and already known ones have to be optimized. She has developed parallel versions of the algorithms using different architectures, methodologies and parallel programming languages. Her research has been funded for the past 10 years through her participation in five national projects; seven regional projects, as well as two European Cost shares and two thematic networks: e-science and CAPAP-H. The researcher is also a reviewer of prestigious journals included in the JCR and of several National Research Agencies. |
Talk title: Global Optimization in Drug Discovery
Abstract: The discovery of new drugs is a very expensive process, frequently taking around 15 years with success rates that are usually very low. New techniques based on principles of Physics and Chemistry were developed about three or four decades ago for the computer simulation (mainly using high-performance computing architectures) of systems of biological relevance. Computational chemistry was later applied for processing large compound databases, and also for predicting their bioactivity or other relevant pharmacologic properties. Using this approach, it was shown that it was possible to use such computational methodology to pre-filter compound databases into much smaller subsets of compounds that could be characterized experimentally. This idea was named Virtual Screening (VS), and it reduces the time needed and expenses involved when working on drug discovery campaigns. Among the most widely used VS approaches, Shape Similarity Methods compare in detail the global shape of a query molecule against a large database of potential drug compounds. Even so, the databases are so enormously large that, in order to save time, the current VS methods are not exhaustive, but they are mainly local optimizers that can easily be entrapped in local optima. It means that they discard promising compounds or yield erroneous signals. In this work, we propose the use of efficient global optimization techniques, as a way to increase the quality of the provided solutions. In particular, we introduce OptiPharm, which is a parameterizable metaheuristic that improves prediction accuracy and offers greater computational performance than most known VS algorithms. OptiPharm includes mechanisms to balance between exploration and exploitation to quickly identify regions in the search space with high quality solutions and avoid wasting time in non-promising areas.
![]() |
Jan W. Owsiński is the deputy director for research and a research scholar at the Systems Research Institute, Polish Academy of Sciences. He also lectures at the Warsaw School of Information Technology and Management, is the Executive Editor of the quarterly Control and Cybernetics, Editor of Modern Problems in Management, a journal of Warsaw School of Information Technology and Management, Secretary General at the Polish Operations and Systems Research Society. Published altogether more than 250 papers and more than 30 edited volumes, the latter largely in English. His scientific interests include methods of data analysis and AI (primarily cluster analysis and preference aggregation) with applications mainly in economics, ecology and biology; knowledge processing and management (data-information-knowledge feedback system); e-Government, e-Economy, e-Society; economics of the transition countries; environmentally, socially and economically sustainable development, and life quality; transport and logistics – network optimisation. More information about prof. dr. Jan W. Owsiński. |
Talk title: Reverse Clustering, Classification And Extrapolation: Some Basic Interpretations
![]() |
Prof. Dr. Álvaro Rocha
Álvaro Rocha holds the title of Honorary Professor, DSc in Information Science, PhD in Information Systems and Technologies, MSc in Information Management, and BCs in Computer Science. He is a Professor of Information Systems at University of Coimbra, researcher at CISUC (Centre for Informatics and Systems of the University of Coimbra) and a collaborator researcher at LIACC (Laboratory of Artificial Intelligence and Computer Science) and at CINTESIS (Center for Research in Health Technologies and Information Systems). His main research interests are Information Systems Planning and Management, Maturity Models, Information Systems Quality, Online Service Quality, Intelligent Information Systems, Software Engineering, e-Government, e- Health, and Information Technology in Education. He is also President of AISTI (Iberian Association for Information Systems and Technologies), Chair of IEEE Portugal Section Systems, Man, and Cybernetics Society Chapter, Editor-in-Chief of JISEM (Journal of Information Systems Engineering & Management) and Editor-in-Chief of RISTI (Iberian Journal of Information Systems and Technologies). Moreover, he has acted as Vice-Chair of Experts in the Horizon 2020 of the European Commission, as Expert in the Ministry of Education, University and Research of the Government of Italy, and as Expert in the Ministry of Finance of the Government of Latvia. |
Talk title: Maturity Models for Medical Informatics Management - A Data Analytics Maturity Model
Abstract: In the last five decades, maturity models have been introduced as reference frameworks for Information System (IS) management in organizations within different industries. In the healthcare domain, maturity models have also been used to address a wide variety of challenges and the high demand for hospital IS (HIS) implementations. The increasing volume of data is exceeded the ability of health organizations to process it for improving clinical and financial efficiencies and quality of care. It is believed that careful and attentive use of Data Analytics in healthcare can transform data into knowledge that can improve patient outcomes and operational efficiency. A maturity model in this conjuncture, is a way of identifying strengths and weaknesses of the HIS maturity and thus, find a way for improvement and evolution. This talk presents a proposal to measure Hospitals Information Systems maturity related with Data Analytics. The outcome is a maturity model, which includes six stages of HIS growth and maturity progression.